Mobile Manipulator
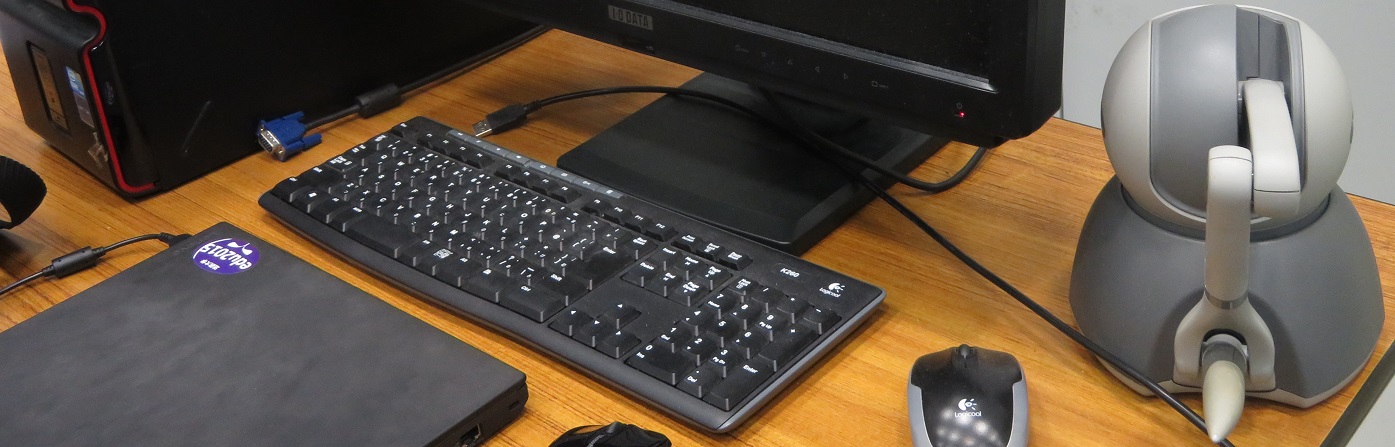
Recycling of printed circuit boards by robot manipulator: A Deep Learning Approach
In recent years, as the birthrate declines and the population ages, manpower shortages in industrial and nursing care settings have become increasingly serious and are widely recognised as a social issue. According to the ‘Robot Industry Market Trend Survey Results’ published by the Ministry of Economy, Trade and Industry in 2013, the robot market is expected to reach 5.3 trillion yen in 2025 and 9.7 trillion yen in 2035. The market is expected to expand remarkably, especially in the service sector other than the manufacturing sector. In addition, with the rapid development of the online sales market, the shortage of manpower at logistics sites is becoming even more pronounced, and efforts are being made to realise labour-saving and manpower-saving measures. Against this backdrop, a public-private council has been established with the aim of using mobile robots for delivery, and deregulation, including legal amendments, is also being actively considered. From these developments, it is clear that the introduction of mobile robots is accelerating not only in the manufacturing industry but also in various other fields such as logistics, retail and nursing care, and that mobile robots are making a significant contribution to improving efficiency, reducing costs and eliminating labour shortages. The efficient execution of work by mobile robots depends on highly accurate self-position estimation technology. In recent years, self-position estimation technology has evolved considerably, and with highly accurate sensors and algorithms, robots can accurately determine their own position even while on the move. In previous studies, methods for simultaneous position estimation using RGB-D cameras and Laser Range Finder have attracted attention. However, these methods are vulnerable to lighting conditions and dynamic environments, and their accuracy decreases when the environment changes. Recently, self-position estimation using deep learning has been proposed to improve the accuracy even in complex environments. In particular, SLAM using deep learning provides a more robust system, but further research is needed for practical use. However, in the diverse work environments faced by mobile robots, not only position estimation but also accurate object picking is an important issue. It is difficult for a mobile robot alone to grasp an object precisely, especially in pick-and-place operations. On the other hand, camera-equipped manipulators are capable of high-precision recognition and grasping operations, but their limited working range makes it difficult for them to handle a wide range of tasks. The integration of high-precision self-positioning and object grasping technologies is essential for the construction of more flexible and effective robot systems. In this study, a ‘mobile manipulator’ that integrates a mobile robot and a manipulator was developed. This system introduces a self-position estimation technology based on Contrastive Learning (CL), which is combined with object recognition technology based on Faster R-CNN to realise highly accurate and efficient picking work in an indoor environment.
Students